In the past few years, the field of quantum machine learning has gained much attention because of its prospective advantages for machine learning calculations by means of quantum parallelism and entanglement. Among other topics, quantum autoencoders—counterparts to classical autoencoders, which are neural networks that can learn efficient codings of large datasets—are being explored due to their potential in efficiently compressing quantum information.
After proving that an ideal quantum adding device that is able to produce a superposition of two unknown quantum states is forbidden by the laws of quantum mechanics, a group of researchers from the University of the Basque Country UPV/EHU and Shanghai University have analyzed the possible implementation of approximate quantum adders capable of producing superpositions to a certain fidelity.
A previous study proposed that approximate quantum adders may be optimized with a classical machine learning algorithm via genetic algorithms, and were employed to compress quantum information to a certain fidelity by means of an approximate quantum autoencoder.
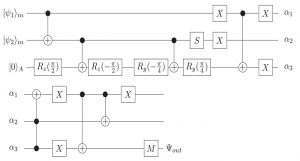
Quantum circuit for the basis adder U, which is equivalent to the operation U in Equation 3. The basis adder provides a fidelity 1 for the addition of the computational basis states and approximately adds their superpositions.
In their recent study, the team, which included Professor Enrique Solano and Professor Xi Chen, produced a quantum autoencoder in the Rigetti cloud quantum computer via quantum adders based on the initial work by Lamata. This pioneering experiment may prove useful in efficiently compressing quantum information and could be important in emerging photonic setups.
“We have implemented a quantum autoencoder via quantum adders in the Rigetti cloud quantum computer,”says Dr. Lamata. “This kind of approach may be useful in the future for efficient compression and communication of quantum information, possibly in the framework of quantum satellites and quantum drones”.