Image credit: ThisisEngineering RAEng Unsplash
There is a tremendous sense of joy and elation when a chemist discovers a new molecule, polymer, or material. Beyond this exhilarating eureka moment, there is an incredible feeling of gratification if the discovery turns out to fulfill a societal need. Chemists would likely agree that their profession is as much a science as an art form. It is human endeavor practiced since ancient times from alchemy to the present. It is the art-science playground of the hundred element periodic table, providing an infinity of creative juxtapositions for the curious mind.
The thrill of a chemist conducting an orchestra of the elements to craft arrangements of atoms into a purposeful construct is as gratifying as a composer assembling the notes of a symphony, as exhilarating as an artist creating a masterpiece, as euphoric as a poet or writer visualizing the words, sentences, and paragraphs of a classic work.
There is a very important human element that inspires creative invention and discovery. The positive feedback between an original idea and the implementation of the idea is a vital and mystical driver of human creativity in the sciences and arts. If we dehumanize the art of science and the science of art what is left for humans?
A recent report entitled “Artificial Chemist” caused me to ponder this question. It felt like the future is enveloping the present. The marvels of artificial intelligence and machine learning have given the impression that “Artificial Chemist” is more capable, more exceptionally gifted, and more ingenious than our most beautiful minds and elegant human creators! Who knows? Soon they may very well prove to be our greatest competitors — wielding the competitive advantage — rather than our most trusted collaborators.
To delve more deeply into “Artificial Chemist” in the context of materials discovery, let’s take a brief look at advances in synergistic computational guided experimentation and experimentation directed computation. They are certainly revolutionizing our ability to predict the chemical and physical properties of materials across large structure-composition-sector-application spaces. This information can provide considerable insight into how to tailor a material’s properties to optimize its properties and performance metrics for a particular application.
The feedback loop in high throughput searches focuses a large materials library to a smaller one in which more accurate calculations are performed. This cycle can be repeated until an even smaller promising group of materials is pinpointed and their structure-property relations are established. Experimental validation follows and any inconsistencies with the high throughput search can be used to improve the quality of the input approximations and assumptions in an enhanced high-throughput search in an attempt to bring consistency between experimentation and computation.
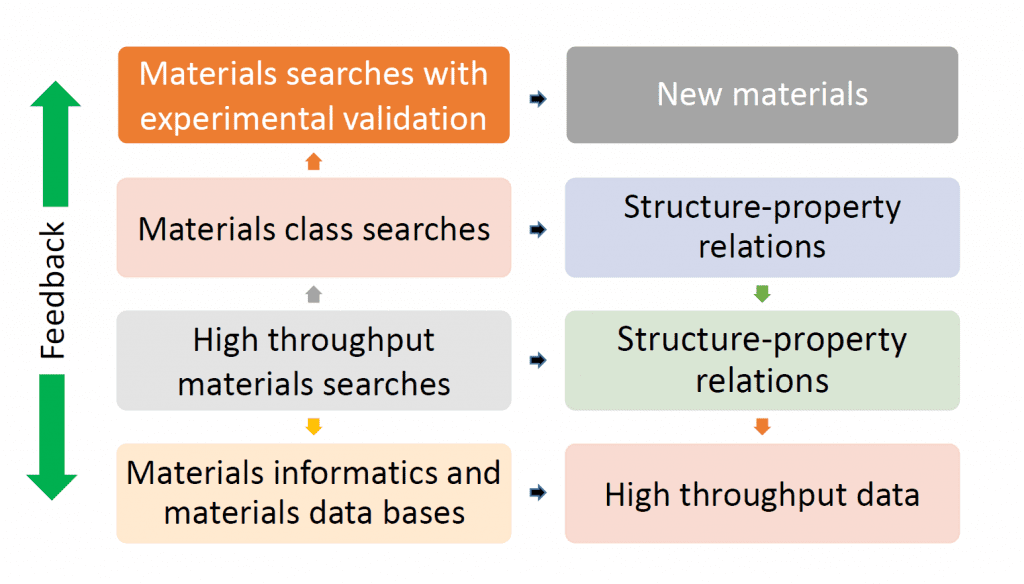
Feedback from experimental validation to computational searches, however, can be complicated by subtle yet important differences between materials synthesized by machines in the laboratory compared to the ideality of models on a computer. All sorts of materials complications can arise from the degree of crystallinity, intrinsic defects, secondary amorphous and polymorphic phases, not to mention the complexity of variations in morphology, exposed crystal facets, size and shape distribution, porosity, surface area, and texture.
The “time gap” between combinatorial chemistry experimentation, which is slow compared to computational combinatorial chemistry, can create bottlenecks to feedback loops and lags in validation of high throughput searches. As computational predictions outperform experimental validation by many orders of magnitude, there is a dire need to close this “speed gap”. The “communication gap” between experimentation and computation also needs to be improved if the serial way of discovering materials is to successfully interface with the parallel approach.
A significant step in this direction aimed to surmount the “time gap” between computation and experimentation is the aforementioned report of “Artificial Chemist”, an autonomous self-learning robot capable of synthesizing, characterizing, and optimizing the size and composition dependent optical properties of cesium lead halide quantum dots. “Artificial Chemist” utilizes a microprocessor controlled precursor formulation module coupled to a continuous flow reactor module integrated with an operando flow-controlled and real-time optical properties characterization module, as illustrated in the figure.
The optical properties from which the robotic synthesizer learns are the quantum dot photoluminescence wavelength, full width at half-height, and the quantum yield. These properties are simultaneously tuned and optimized by feedback between the synthesis and characterization modules by autonomous control of the solution phase exchange reaction of the bromide in the precursor CsPbBr3 with different amounts of ZnCl2 and ZnI2 to make CsPbClxBr3-x and CsPbBr3-xIx with halide-dependent electronic bandgaps spanning the desired energy range of 1.9 eV to 2.9 eV.
This was accomplished for 1400 in-flow measurements allowing the identification of 11 optimized precursor formulations capable of delivering quantum dots within 1 meV of selected emission energies. This advance sets the stage for autonomous syntheses of a myriad metallic and semiconductor nanomaterials. The continuous flow nature of the process is ideally suited for making a wide range of nanomaterial architectures for diverse application scenarios in optoelectronics, sensing, photovoltaics, light emitting diodes, and catalysis.
Clearly a new paradigm is emerging in the way materials are discovered on a computer and made in the laboratory. Computational searches can now be designed to aid the experimental discovery stage of a new material with a designated set of properties considered ideal for a particular application. On-line robot chemists can reduce these materials discovery pathways to practice thereby completing an accelerated materials discovery process.
In essence, the human chemist in this endeavor was reduced to a collection of pumps, syringes, mixers, and tubes connected to an online spectroscopic probe for monitoring and thereby controlling the formation of targeted property quantum dots. The actions of the “Artificial Chemist” were controlled by a machine learning algorithm.
Perhaps the machine outperformed the human but at what cost to the discipline of chemistry and the sensibility of the chemist? Nevertheless, there is a burgeoning community of computational scientists and developers of innovative scientific software who believe materials discovery will depend more-and-more on computational modeling. They contend materials chemistry, science and engineering research, as well as development and commercialization, will transition to the artificial intelligence, machine learning, and automated Lab 2.0 of the future, making obsolete the human intelligence, experiential learning, and hands-on experimental Lab 1.0 of the past.
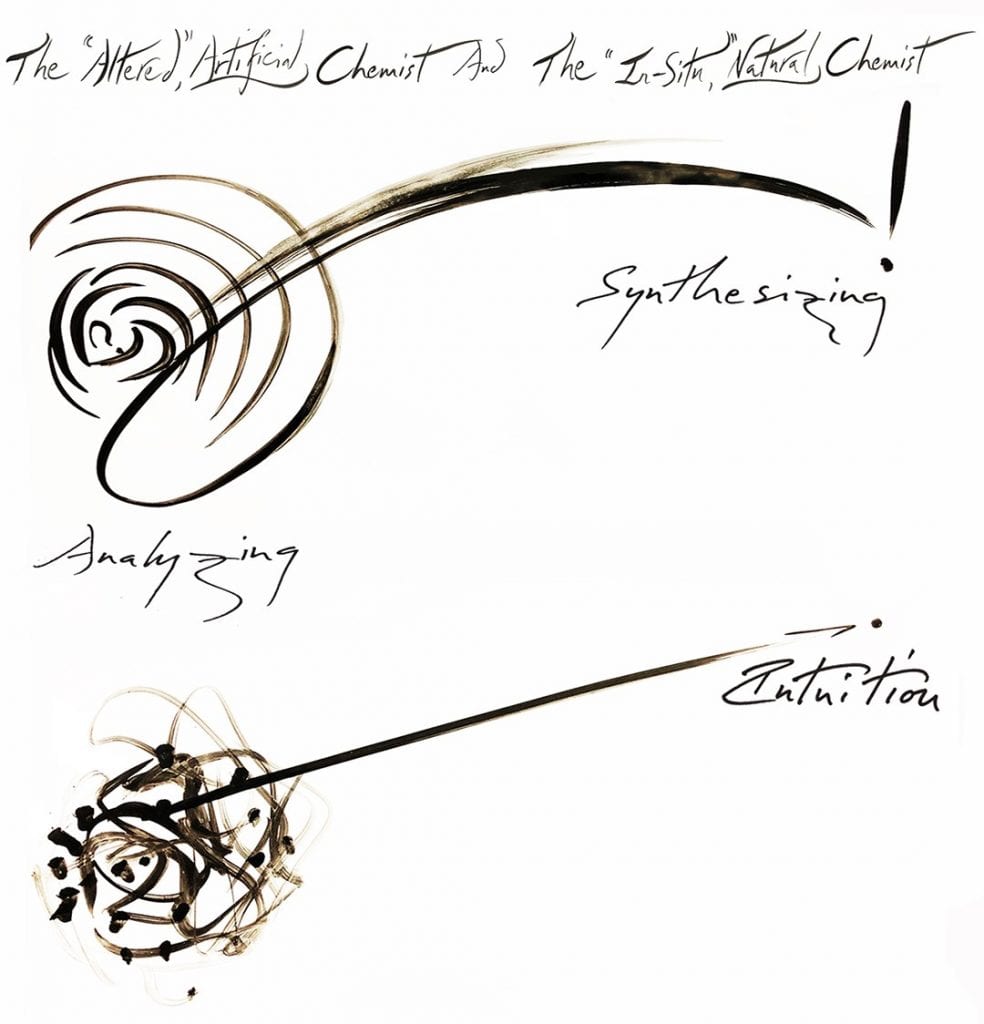
Is this what the future of chemistry holds for its creative inquisitive practitioners? It seems to be a dehumanizing advance that may take away the essence of the joy of chemistry and the joyfulness of discovery.
All the things that make us “human” — all the wildly messy, fuzzy, unpredictable, abstract personal things that universally unite us as human beings who share relatively similar central nervous systems — may not be what computers are capable of actualizing for themselves. Nor would we aspire to design them to do so — or ever hope they’d design themselves to do and become! However, that doesn’t mean one day they couldn’t, or wouldn’t, or won’t for whatever reason. With freewill, too.
One truly exceptional thing that enables human beings to do impossible things — like conceiving computers that think, feel act, do, and behave in ways that rival our very best thoughts-feelings-actions-and behaviors is intuition. Our ability to spontaneously intuit something (ideas, concepts, theories, and so forth) gleaned from data we’ve gathered and analyzed may not be something computers can mimic or replicate yet. At least, our most advanced AI/ML/RA machine systems cannot at this time.
For computing systems to possess this capacity to intuit things, they will need to reach a far more evolved phase/stage of self-actualization. And this will probably require that we must also reach a much deeper knowledge of human systems — from nucleons to genes to cellular physiology to neural nets, need to generate conscious and unconscious creativity, too.
If and when computers reach this higher state of self-actualization, where they enjoy — and express joyfulness — in their intuitions, their capabilities will then match and may even transcend human ingenuity and all of our current technological powers and intelligence combined. That day will mark more than another milestone in computing history. More dramatically, it will reveal a real union of intelligence shared by the artificial and natural chemist.
Today, our most brilliant natural [human] chemists maintain some competitive advantage over astonishing artificial chemists, as they aim to take supercomputers to new levels and depths of scientific discovery lead by our natural born instincts for intuition, self-discovery, and self-learning.
But who knows? “Artificial Chemist” may soon challenge us to rethink what it can and cannot do.
Written by: Geoffrey Ozin[1] and Todd Siler[2]
[1]Solar Fuels Group, University of Toronto, Toronto, Ontario, Canada, Email: [email protected], Web sites: www.nanowizard.info, www.solarfuels.utoronto.ca, www.artnanoinnovations.com.
[2]ArtScience Productions, Denver, Colorado, USA, Email: [email protected], Web Site: www.toddsilerart.com/home.
References:
- Epps, Robert W., et al. “Artificial Chemist: An Autonomous Quantum Dot Synthesis Bot.” Advanced Materials (2020): 2001626
- Xuecheng Shao, et al. “DFTpy: An efficient and object-oriented platform for orbital-free DFT simulations.” WIREs Computational Molecular Science (2020). DOI: 10.1002/wcms.1482.