Image credit Chi-Yuan Yang and Matteo Massetti. Reprinted with permission.
Over the last couple of decades, researchers from all over the world have attempted to build computing systems called neuromorphic devices that emulate the human brain. Unlike conventional computers, the brain is able to compute and store information at the same time, relying on “synaptic plasticity” — the ability to change and adapt to new information through the adjustment of synaptic weights to integrate different stimuli to perform computations.
Conventional, silicon-based circuits mimicking the brain require hundreds of transistors to simulate just a single neuron. Since the human brain contains around 1011 neurons and 1014−15 synapses, scaling up these circuits into a functioning neuromorphic computer is difficult due to issues related to volatility, design complexity, and power constraints.
Other memristor-type technologies, such as those based on filament-forming metal oxides or phase change memory materials, are currently being developed, but they suffer from drawbacks such as high noise, nonlinear response, and high write voltage/current. This complicates the path to achieving interconnectivity, as well as information density and energy efficiency in the computer.
The brain also remains unique in the sense that it has the capability to make new connections where none existed before. While brain-inspired technologies have advanced the field of artificial intelligence by emulating the information processing of biological systems, they fail to emulate the way biological systems interpret the environmental signals and respond to them.
Organic electrochemical transistors (OECTs) have proven to be particularly attractive for neuromorphic computing. However, existing OECT technology still relies on prefabricated circuitry that can be adjusted through supervised or unsupervised learning to accomplish a given task.
To this end, a team led by Simone Fabiano of Linköping University reports using environmental inputs to trigger, for the first time, the controlled growth of an artificial synapse an “evolvable” organic electrochemical transistor. Their findings were recently published in the journal Advanced Electronic Materials.
The channels formed between the device’s synapses were created in situ and in operando through the polymerization of a conductive polymer. The resulting transistor exhibits long-term changes in the channel conductance at a fixed voltage, without the need of conventional relays, which are typically used in other state-of-the-art transistor-based artificial synapses.
The authors explored building various biomimetic circuits based on this evolvable transistor, which grow after the implementation of environmental inputs — for example, temperature and pressure — to achieve higher order computing processes (see the figure below) like self-regulation of synaptic strength and coincidence detection, analogous to afferent sensory signals, which carry nerve impulses from the central nervous system to the brain.
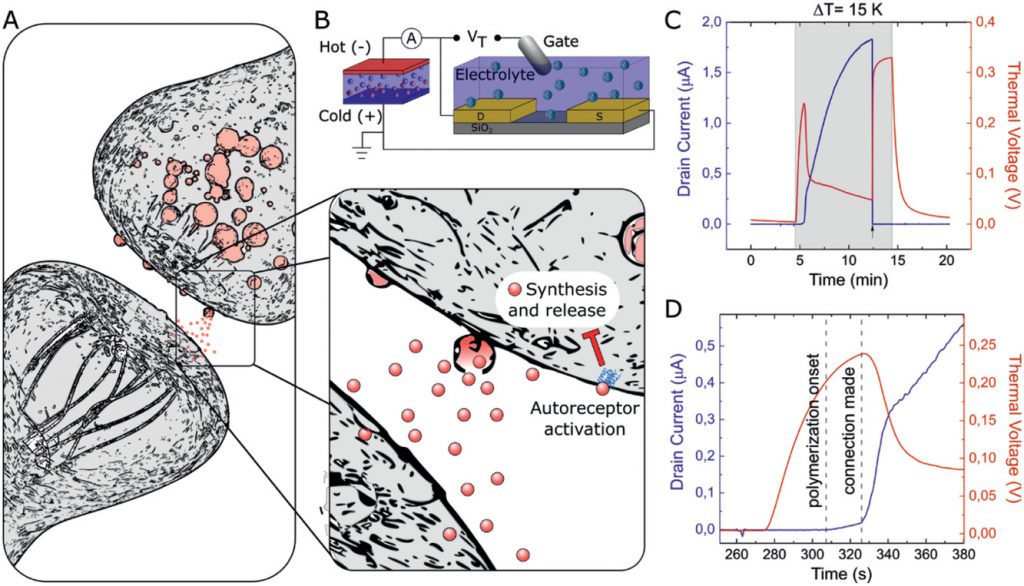
The next challenge will be to scale down the device’s dimensions to reduce the energy consumption and increase the number of devices per unit area. The ability to grow, evolve, and adapt upon training opens the way to the development of self-learning hardware, with unlimited potential in areas such as brain-machine interface, Internet of Things, robotics, and more.
Reference: Jennifer Gerasimov, et al, A Biomimetic Evolvable Organic Electrochemical Transistor, Advanced Electronic Materials (2021). DOI: 10.1002/aelm.202001126