Image credit: Immo Wegmann Unsplash
With all the excitement surrounding artificial intelligence and machine learning and their growing role in materials discovery, I wonder if this emerging strategy for research will be able to transcend the challenges of materials reproducibility that has always confronted the best practices of human intelligence, experiential learning, and hands-on expertise.
According to the American Standard of Testing and Materials, ASTN International, materials reproducibility is ascribed as the precision with which a measurement is made on a material by a particular analytical method under a specific set of test conditions.
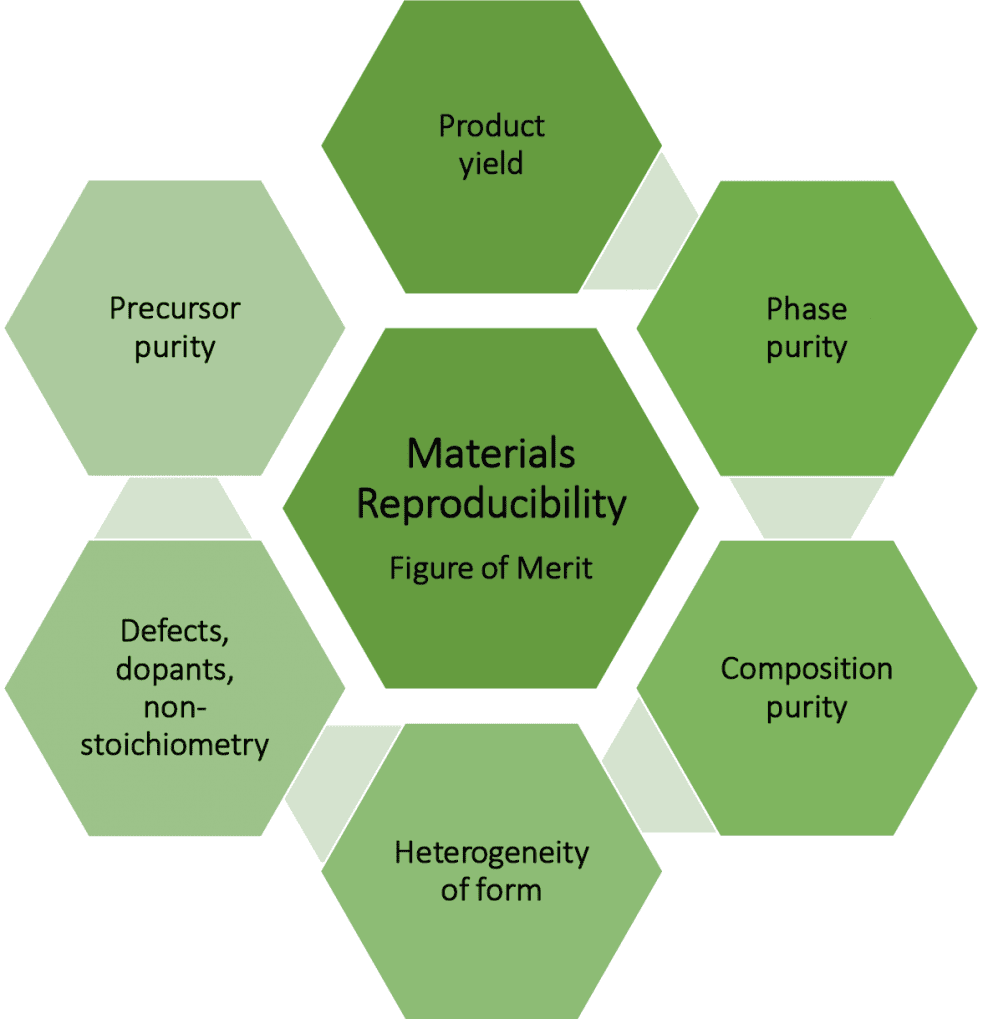
It certainly is a challenge to discover a new material or improve one that already exists, but it is equally demanding to do this repetitively and reproducibly — prerequisites for its success both scientifically and technologically. The complexity of rising to this challenge can be appreciated by what exactly is implied by a metric of reproducibility for a bottom-up synthesis or top-down fabrication of a material (envisioned in the figure).
As the majority of materials in the field of renewable energy are inorganic, the question of reproducibility in this commentary is focused on this class of solids.
Purity is one task in evaluating reproducibility, but at what level? This is an important question that turns out to be dependent on the property of the material and the effect of different levels of purity-induced variability on its performance metrics when integrated in a product, process, or device.
It is important to keep in mind that no matter how inorganic solid-state materials are prepared, most are insoluble in solvents and therefore cannot be purified by crystallization methods akin to most molecular, cluster, and polymeric solids. One often has but a single chance in the preparation of a solid-state material to control its purity, where purity can refer to its composition and phase.
Therefore reproducibility of composition and phase will depend upon a preparation that yields the desired level of elemental and structural purity. This will depend on the degree of purity of precursors used in the preparation and its quantification and reproducibility from batch to batch and supplier to supplier, an often overlooked aspect of a chosen materials preparative pathway.
Criteria for judging the effect of purity will be predicated upon the specific detection limit of the analytical techniques employed to establish an elemental composition and phase purity figure of merit. These metrics are crucial measures of reproducibility of a materials preparation as unintentionally incorporated dopants, defects, non-stoichiometry, impurities, occlusions, and adsorbed species can have dramatic consequences on the physical and chemical properties of a material. For example, an unintended dopant can switch an intrinsic electrically insulating material to become a semiconductor, an unwanted vacancy defect can change a semiconductor into a metal or even a superconductor, and these effects can operate at less than a part per hundred of an adventitious dopant or defect.
An equally important determinant of reproducibility of a material is the heterogeneity of its physical form. The form is a generic descriptor for whether the material is made as a single crystal, a thin film, or nanocrystal. In the case of nanocrystalline materials, one has to be concerned about quantification of the reproducibility of its size, shape, and surface. This is especially true with respect to the heterogeneity of nanocrystal sizes, state of aggregation, interfaces and grain boundaries, and textural porosity. The composition of the material comprising the nanocrystals will control the size range in which quantum size effects prevail and the physical scaling laws that determine the size dependence of the nanocrystals optical, electrical, magnetic, and thermal properties. Reproducibility of the nanocrystal size distribution from synthesis to-synthesis within the same laboratory or between different laboratories is incredibly challenging.
Could an autonomous robot do better than a human operator? Perhaps, because variability might come from a scientist’s skill set/quirks where this could be eliminated in a programmed procedure.
The surface of nanocrystalline materials presents a rather unique hurdle in the quest for reproducibility. It is fair to say that the surface of a nanocrystal presents the largest number of unknowns with respect to reproducible control of its morphology and the occurrence and prevalence of different crystal facets. Making single-size and single-shape nanocrystals bearing a single kind of facet represents the leading-edge of the field of nanochemistry. Normally, one has a distribution of each in the synthesis of a nanocrystalline material, which can blur the effects of quantum confinement and confuse the effects of surface structure and surface area on for example, thermochemical, electrochemical, and photochemical surface chemistry and catalysis, ionic, electronic, and thermal conductivity.
Finally, there is the question of reproducibility of product yield. For molecules and polymers that can be made and purified as single product and phase, the yield is a quantity that in principle can be precisely defined but what do we mean by the yield of an ensemble of nanocrystals with variable sizes and shapes and states of aggregation? Perhaps measured mass yield of the entire distribution adequately defines the reproducibility of a nanocrystal preparative method where each component in the histogram of their size and shape in principle should be quantified as a figure of merit for the reproducibility of the preparation of a material.
Clearly there is much more to materials discovery carried out by humans and machines than just discovery! Is this an aspect of materials discovery by artificial intelligence and machine learning implemented by autonomous robots that can and will outperform us humans?
Written by: Geoffery Ozin
Solar Fuels Group, University of Toronto, Toronto, Ontario, Canada, Email: [email protected], Web sites: www.nanowizard.info, www.solarfuels.utoronto.ca, www.artnanoinnovations.com